Just Add $100 More: Augmenting Pseudo-LiDAR Point Cloud
for Resolving Class-imbalance Problem
Neurips 2024
- 1Korea University
- 2NAVER LABS
Abstract
Typical LiDAR-based 3D object detection models are trained with real-world data collection, which is often imbalanced over classes. To deal with it, augmentation techniques are commonly used, such as copying ground truth LiDAR points and pasting them into scenes. However, existing methods struggle with the lack of sample diversity for minority classes and the limitation of suitable placement. In this work, we introduce a novel approach that utilizes pseudo LiDAR point clouds generated from low-cost miniatures or real-world videos, which is called Pseudo Ground Truth augmentation (PGT-Aug). PGT-Aug involves three key steps: (i) volumetric 3D instance reconstruction using a 2D-to-3D view synthesis model, (ii) object-level domain alignment with LiDAR intensity simulation, and (iii) a hybrid context-aware placement method from ground and map information. We demonstrate the superiority and generality of our method through performance improvements in extensive experiments conducted on popular benchmarks, i.e., nuScenes, KITTI, and Lyft, especially for the datasets with large domain gaps captured by different LiDAR configurations. Our code and data will be publicly available upon publication.
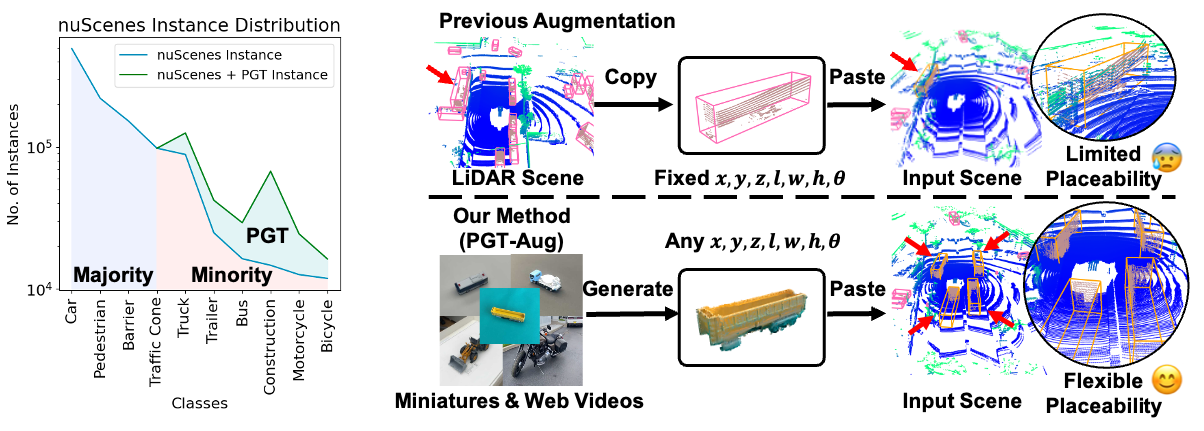
Citation
If you want to cite our work, please use:
@article{DBLP:journals/corr/abs-2403-11573, author = {Mincheol Chang and Siyeong Lee and Jinkyu Kim and Namil Kim}, title = {Just Add {\textdollar}100 More: Augmenting NeRF-based Pseudo-LiDAR Point Cloud for Resolving Class-imbalance Problem}, journal = {CoRR}, volume = {abs/2403.11573}, year = {2024}, url = {https://doi.org/10.48550/arXiv.2403.11573}, doi = {10.48550/ARXIV.2403.11573}, eprinttype = {arXiv}, eprint = {2403.11573}, timestamp = {Mon, 08 Apr 2024 18:24:51 +0200}, biburl = {https://dblp.org/rec/journals/corr/abs-2403-11573.bib}, bibsource = {dblp computer science bibliography, https://dblp.org} }
Acknowledgements
The website template was borrowed from Michaƫl Gharbi, MipNeRF360, and 2DGS.